From the NILU Annual Report 2017: During the last decade, the need for information and data has exploded, and this “data revolution” has not stopped short of science. In environmental research, data have become more and more important – to the point that scientists now even know what your fireplace looks like.
– Data collection is the key step in most environmental research fields. When developing high-resolution emissions of both air pollutants and greenhouse gases, the most crucial part is getting high quality data, explains Susana Lopez-Aparicio, senior scientist at the Urban Environment and Industry Department at NILU.
Wood burning data challenge
One of the biggest challenges is the characterisation of emissions from wood burning for residential heating. In Norway, wood burning is an important source of domestic heating, associated with a strong tradition of cosiness. There are approx. 2.5 million wood burning stoves and over half of them are presumed to be in regular use. Wood burning in residential houses does not only provide a warm home, it is also one of the main contributors to harmful air pollutants (e.g. particulate matter-PM, polycyclic aromatic hydrocarbons-PAHs) and an important source of black carbon, a short-lived climate pollutant.
Lopez-Aparicio explains further: – When we want to characterise emissions of wood burning for residential heating, we need information about the wood consumed in the household, the type of stove the wood is burned in (e.g. fireplace, new or old ovens), the time period when wood burning occurs, and the type of dwelling; this indicates the height where emissions enter the atmosphere. When working at city scale, scientists need information at both high spatial and temporal resolution, and those data are rarely available.
The wisdom of the crowd
In the iResponse project (http://iresponse-rri.com), funded by the Research Council of Norway, the NILU team has developed and evaluated different methods based on crowdsourcing. The concept of crowdsourcing is based on collecting ideas, data and/or services from a large group of people by using information and communication technologies (ICTs). It has been defined as the new “paradigm of knowledge creation”. One of the aims of iResponse is to address the challenges and opportunities associated with data collection methods to improve the understanding of emissions from wood burning for residential heating.
– In iResponse, we used two different approaches for data collection, explains Lopez-Aparicio, who coordinates the project. – The first method is based on citizen participation, and the second one on automatic data mining from online portals.
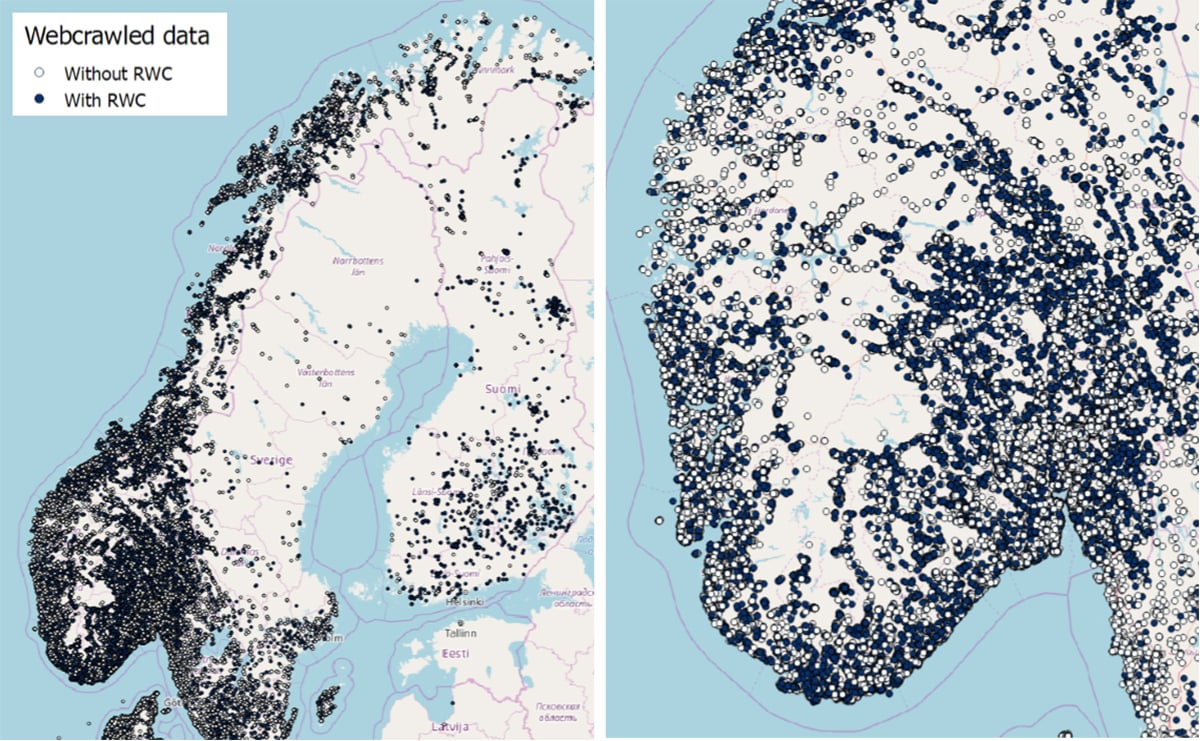
Citizen participation
Lopez-Aparicio and her team designed and tested two methods based on citizen participation. Both aimed to collect geo-localised data on wood consumption per citizen, the type of appliance they use for wood burning, and the time they burn wood.
The first method was based on an existing tool for collecting citizens’ insights of their immediate environment based on geographical information systems (Maptionnaire; https://maptionnaire.com). Case studies were carried out in Oslo, Akershus, Sarpsborg and Fredrikstad, resulting in around 1 000 geo-localised responses with information about the respondents’ wood burning activities and their perception of the environment. For the second method, a specific tool was designed to collect data about wood burning activities, and in return provides the participants with feedback regarding the economic cost and environmental impact associated with their activities.
– We identified important challenges in data collection based on citizen participation, such as volunteer engagement, data quality and sample representativeness, explains Lopez-Aparicio. – It requires a significant amount of resources to achieve a representative participation rate, and obtaining good quality data for use in environmental research is quite challenging.
Automatic data mining
When developing an emission inventory for residential heating, some of the main questions are where the wood burning appliances are and in what type of dwelling. Knowing this, the team is able to identify wood burning potentials for specific areas. The iResponse project developed a method for automatic data mining from online portals where this information is available.
– We designed a so-called “web-crawling” method, says Lopez-Aparicio. – It retrieves the geographical position of the dwellings announced on Finn.no, the Norwegian classified advertisements website that covers the bulk majority of real estate transactions, and pictures from the dwellings’ inside. The announcements give detailed descriptions of the properties, including the available heating system (e.g. heat pump, district heating, wood stove) and the type of dwelling (e.g. detached house, apartment, cabin).
Using machine learning to classify wood stoves
The NILU team developed a model for image recognition and classification based on machine learning. Based on the images collected from Finn.no, the model identifies the wood burning appliances and classifies them with a precision of 81%, 85% and 91% for open fireplaces, old stoves and new stoves, respectively.
The data collected through webcrawling was a crucial piece of information in the development of a model to estimate high resolution emissions from wood burning. This is performed in the MetVed project, financed by the Norwegian Environment Agency and led by Lopez-Aparicio. Here, the analysis of data from different sources has showed the importance of data availability, and the need for more open data.
– Our work clearly demonstrates the potential of such methods to obtain data with high level of detail crucial in the development of emission inventories at urban scale, concludes Lopez-Aparicio.